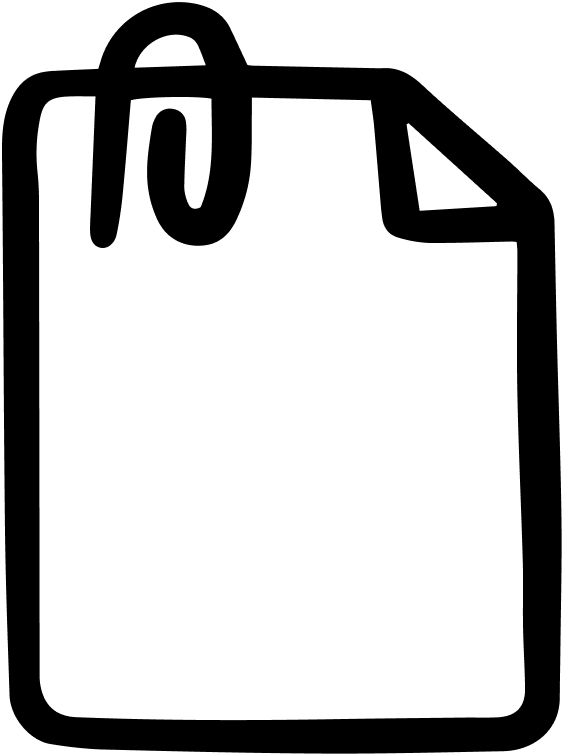
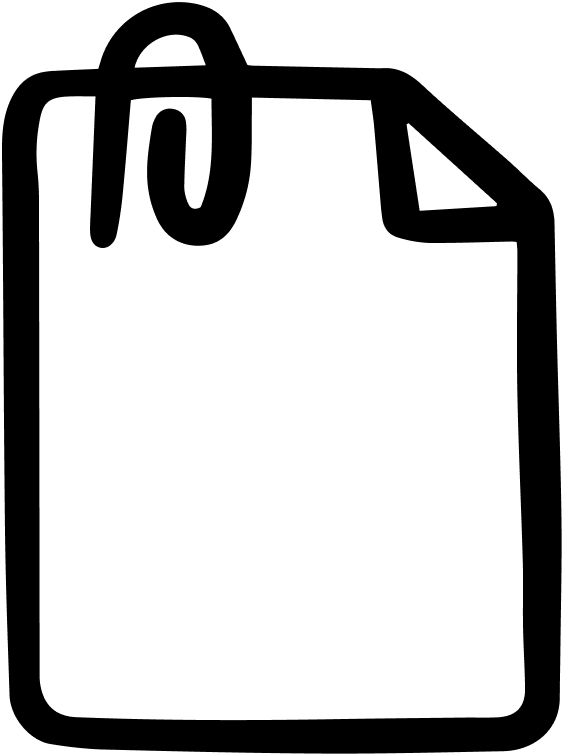
I do a review of the existing studies.
When I’m researching a topic, I start by doing an evidence review — in other words, trying to read a large number of papers (studies) on a topic to get an overall sense of the literature. For example, for a big question like “What is the impact of breastfeeding on short- and long-term health?” I might review 200 papers on the topic. For a smaller question like “Can I do cold plunges while pregnant?” there might be only four or five total.
I find these papers using a combination of Google Scholar searches, searches in PubMed, and, in many cases, referencing UpToDate, a platform designed for doctors.
I often use a form of a “snowball” search, where I read papers, find the papers they cite, then find the papers they cite, and so on. I also work to identify any meta-analyses on the topic, which are essentially summaries of multiple studies that pull out statistical trends.
At this point, I usually have a good sense of what the literature overall says. I then dive more deeply into the papers that are most important in the literature (the ones that are the most highly cited and published in the most highly respected journals), to try to understand in depth what they do, what their limitations might be, and whether some are more compelling than others.
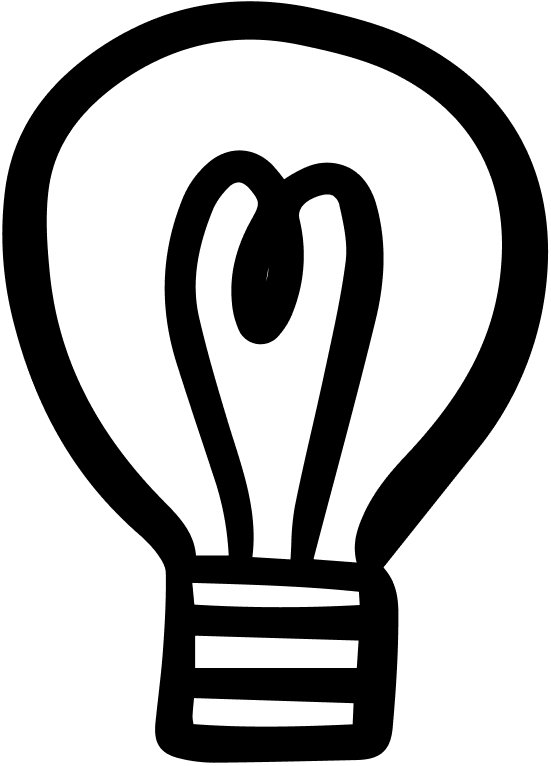
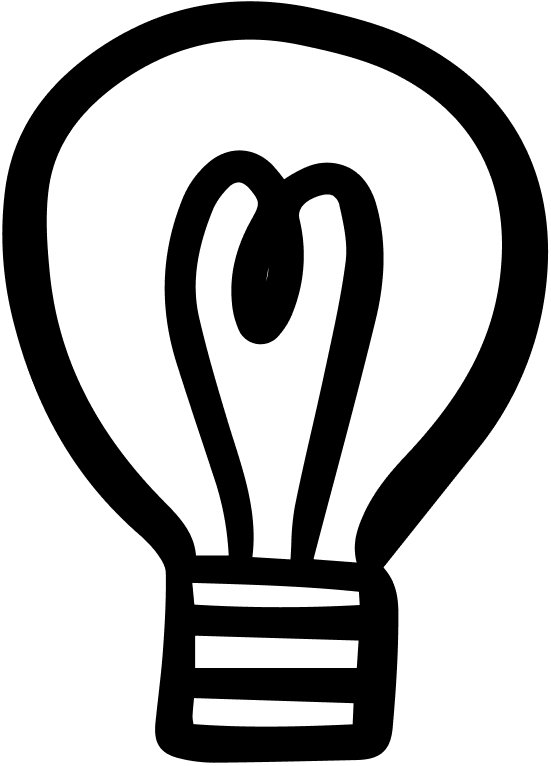
I pick one or two studies to discuss in my writing.
After reviewing the studies, I sit down to write. Here I will often pick one or two studies to highlight as examples.
Sometimes this is because one or two of the studies are much better than the others and deserve all of the focus. In other cases, there are many studies that show basically the same thing and I’ll choose an example that I think represents the overall literature. This lets me explain the details of a single study so people really understand, but also represent the overall picture of what’s in the data.
A good example of this approach is this piece on diets and ADHD. In that case, there are a number of similar studies that look at the impact of a strict elimination diet on ADHD symptoms in children. I chose to talk through the details of one of them — the largest, and the one I thought was most carefully done — as an example of the overall literature.
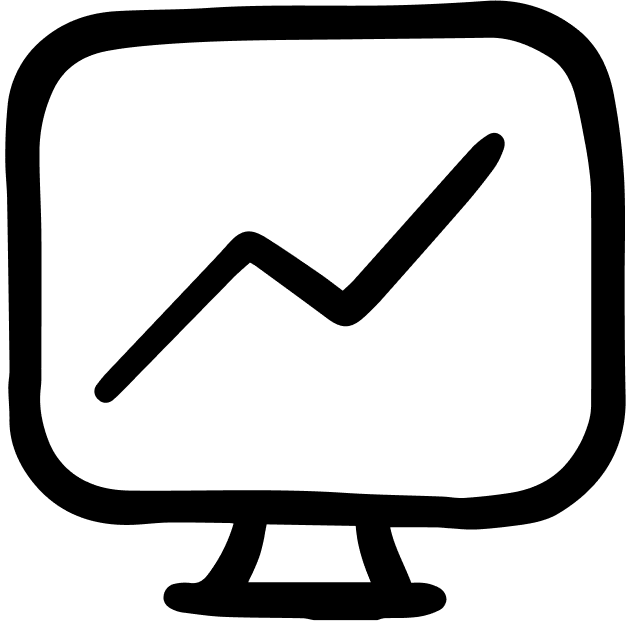
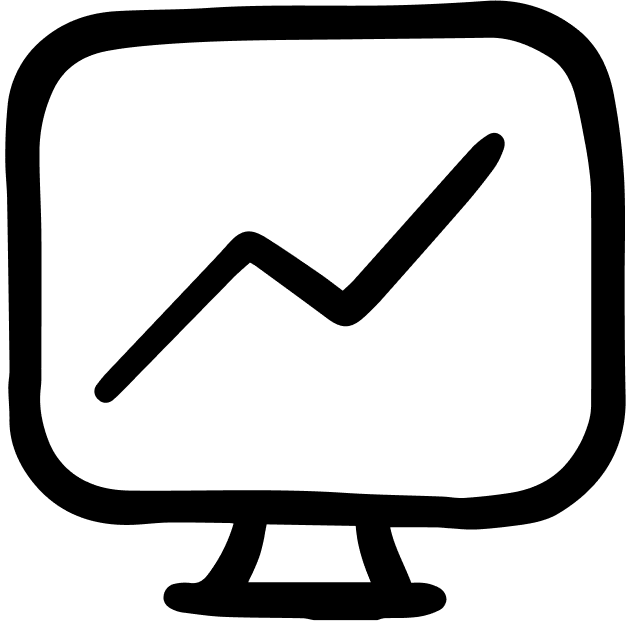
I look for “nice” studies.
Nice is not a technical term, but I do like it. Essentially, I mean “high quality.”
One key element in study quality is whether it shows causation (“x causes y”), rather than a correlation between two connected but unrelated things. This comes up all the time!
To find causation, I’m looking for studies that have randomized data, so we can be confident that the groups of people we are comparing are otherwise similar. You can also find this in studies that use a change in policy or some other source of randomness (this study on paying families to have babies is a good example).
A second element of study quality is size. Bigger studies are on average better. Studying the entire population of Norway is better than a survey of 11 people in New Jersey. (There are exceptions, though, especially in understudied areas of women’s health — I once found an undergraduate senior thesis on breast milk looking at six women that was quite useful and, disappointingly, hadn’t been replicated.)
A final element, especially in medical outcomes, is that it’s much better if we have objective measures than subjective ones. This means, basically, anything that can be measured without asking individuals questions — so things like hospital record data and test scores. These objective measures all increase the validity of the conclusions.
There are exceptions to all of these: small studies, those without randomization, and those with self-reported outcomes that are compelling and that I often rely on. But on average, the considerations above are important.
One thing that often isn’t important is recency. Just because something is newer does not mean it’s higher-quality. You could easily assume that when there’s a headline about a new study that the science has shifted. However, often a new study isn’t a better one, and reviewing what has come before is crucial.
Also, a quick side note here: As a reader, you will often have access to only the abstract (a short summary) of the paper. From that, you can pick up some of the points above, but not all of them. Looking at whether a study is randomized is helpful, and I’d recommend doing that. But unfortunately, it can be hard to go much deeper than that without full paper access and the training to know what to look for.
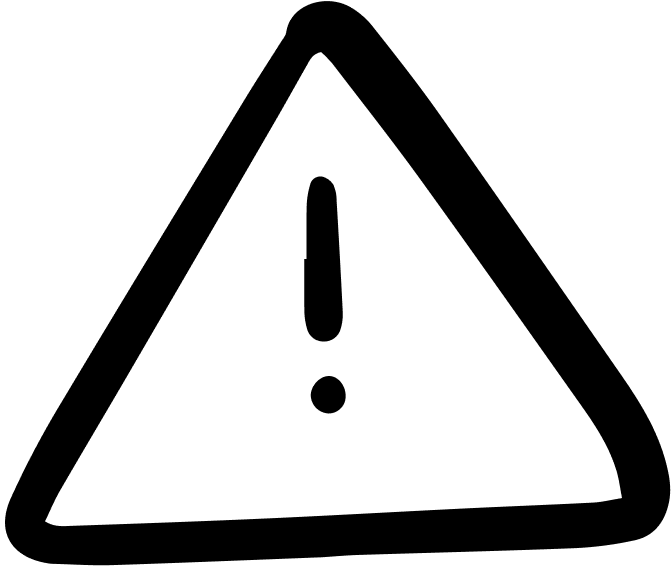
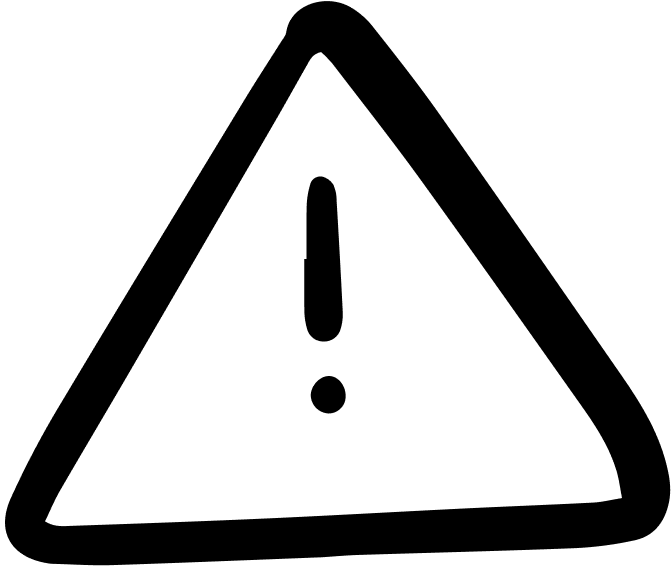
I scrutinize the studies that aren’t randomized.
I am typically far more skeptical than many other people about studies that try to show causation without any randomization (whether by design or natural randomization). My view — motivated by much of my academic work — is that differences across individuals or families that the researcher cannot observe often drive results. Whether it is diet or screen time or some other variable, I generally do not think we can learn causal impacts by just comparing people who do one thing to people who do another. Other researchers are often more willing to draw conclusions from this type of data.
A good example of this is the very large literature on the relationship between alcohol and health. In nearly every study on that topic, the groups who consume different amounts of alcohol also differ in many other ways (education, income, other health behaviors). It’s very challenging to attribute differences in health outcomes to the alcohol in particular.
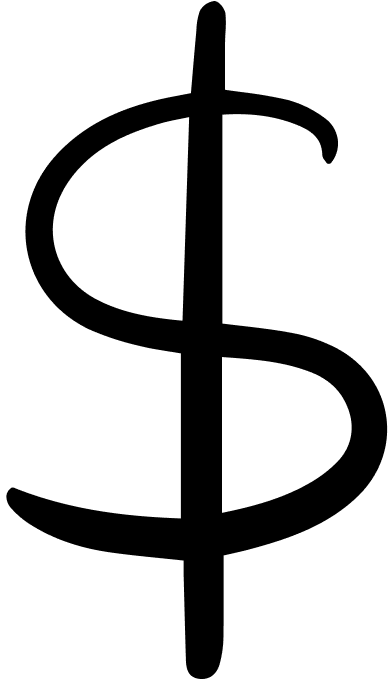
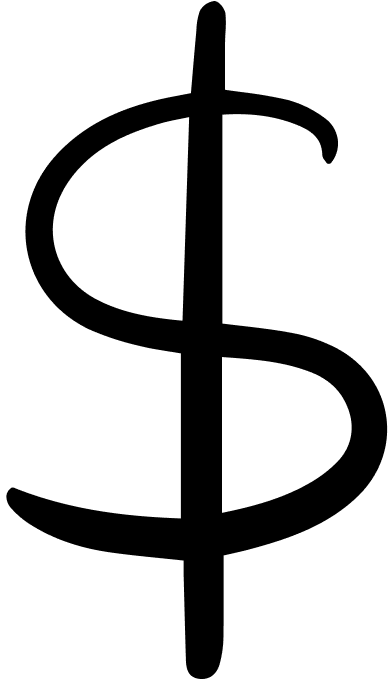
I pay attention to the study funding, but … it’s complicated.
Many studies of vaccines, for example, are funded by the companies that make them. This is by design, and means that those companies bear the risk if the study fails. It’s expensive to run a large vaccine trial, and we wouldn’t want the government to have to bear that cost every time. In the case of something like vaccine or drug trials, there is a lot of external monitoring. So while the company is funding the study, outside parties have a considerable amount of control.
It gets more complicated when you have simpler studies — survey data or observational studies — that are funded by motivated actors. A snack food company that supports a study comparing people who eat its snacks with those who do not and concludes that they improve cognitive performance, for example. I would be skeptical of that.
For me, though, the main reason I would be skeptical is that this isn’t a good way to learn about causality. I would be skeptical of that study even if it were funded by the NIH. In my view, we should be much more concerned with the details of a study and what it actually does than who is paying for it.
If you do want to know who funded a study, that is generally disclosed by the authors somewhere at the start of the paper.
(And no, I do not get paid by authors to talk about their studies.)
What other questions do you have about our methods at ParentData? Is there a study you’d like me to review? Feel free to ask me here anytime.
Ask a Question